
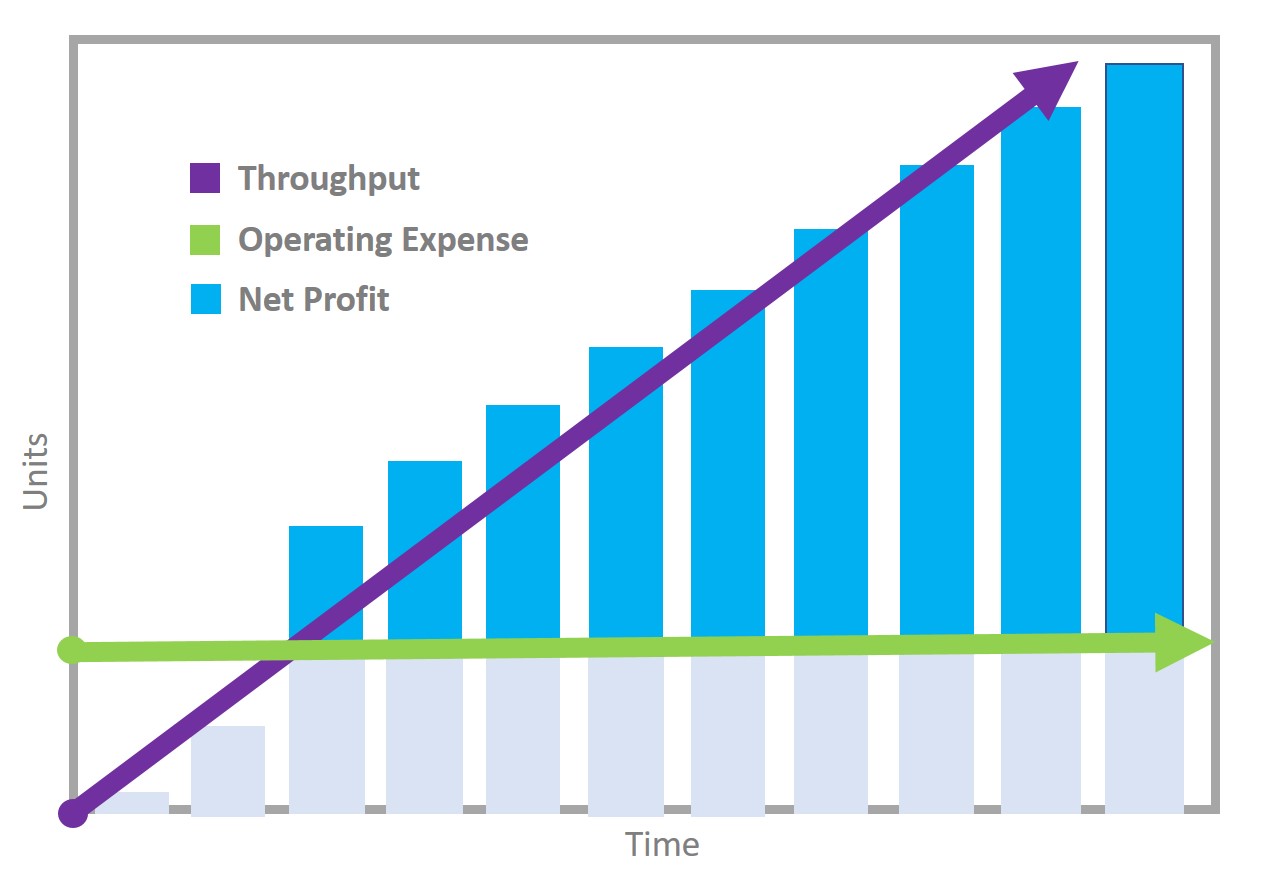
Furthermore, these diverse genetic operations for retrieving the optimal solution to the proposed problem formulation are compared in terms of the estimated precision and time complexity metrics. Through the simulation experiments, the impact of execution of these feasible composite models on the derived two-dimensional Pareto-optimal frontier, the criteria satisfaction ratio and the average spread of individual solutions is extensively investigated. The iterative implementation of the evolutionary genetic algorithm is effectively enforced by considering the comprehensive application of eight disparate combinations of individual creation, selection, mutation and crossover operations. In this work, we employ the meta-heuristic computational method of genetic algorithm for multi-criterion optimization of sigmoidal utility maximization and throughput efficiency. The design of infrastructure-less ad-hoc wireless network with highly dynamic operating conditions can be intelligently modeled by using the artificial intelligence algorithms and machine learning techniques. Facet by multiple app names Throughput-per-minute rate Facet by host-related attributes Query of average duration of a metric Break down average time spent. These attributes are available in addition to the metric-specific attributes listed in the APM metrics table above.With the rapid growth of ad-hoc wireless technology amidst the huge demands of high data-rates, the simultaneous optimization of overall networking throughput and utility-proportional resource allocation is of utmost significance. To understand more about the general structure of metric timeslice data, including some common examples, see Metric timeslice data.
#Throughput with timeslice how to#
Learn how to see all metrics available to you. KeyTransactionName, transactionName, transactionTypeĪpm.Įxternal call response time by transaction type Response time for external calls broken out by external host name
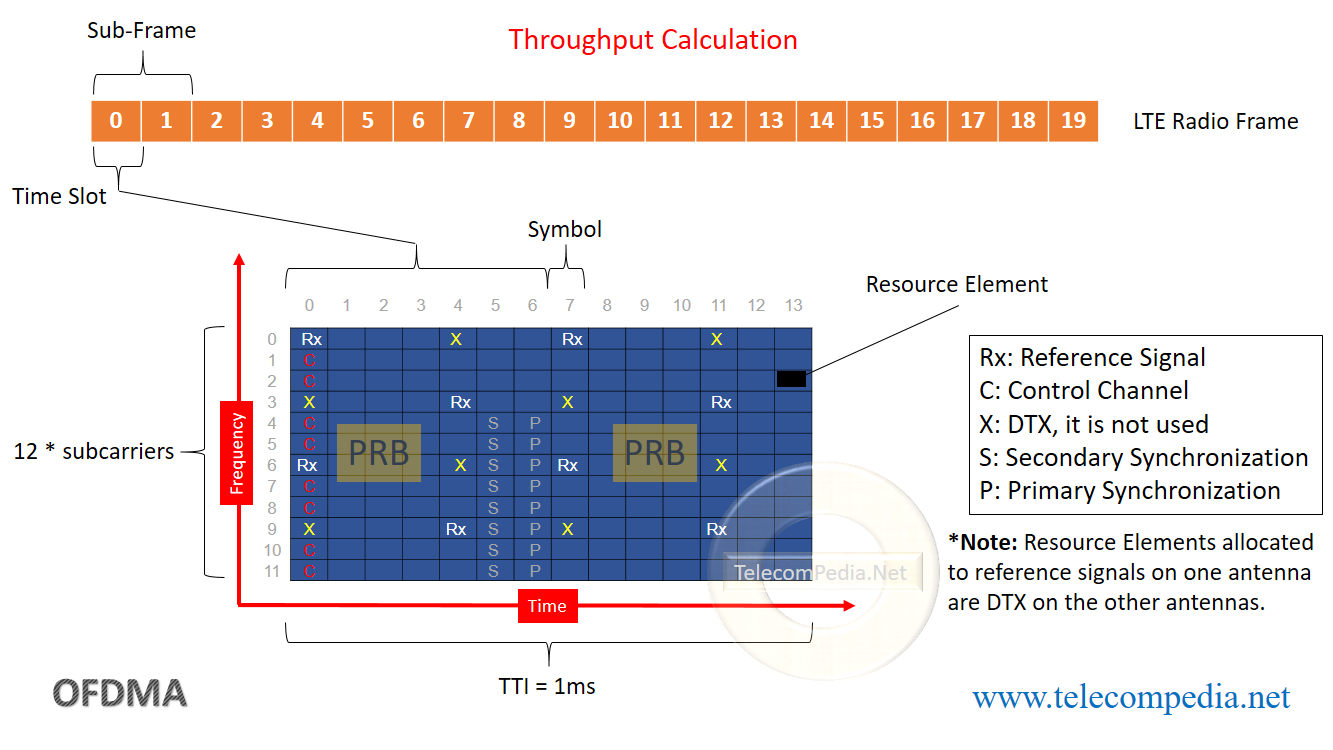
Response time for database calls broken out by table operations Here are how the original APM metric timeslice metrics are converted into dimensional metrics: If you don't see a metric you're looking for in this section, see Generic queries. The conversion of original APM metric timeslice metrics into dimensional metrics that are available for querying is an ongoing process and isn't complete. This optional clause displays the results in a time-based chart.įor general information on NRQL syntax, including FROM, FACET, and TIMESERIES, see Intro to NRQL.įor more queries, see Query examples. Sets the transaction type to web, meaning that background/non-web transactions won't be counted. This query uses entity.guid, but you can also use appId or appName. You can select a single entity's GUID, as shown here, or you can select multiple sources. You must specify at least one data source. Note that you can use other aggregator functions. This query uses the converted metric names. This math generates a count of errors out of a total count of transaction metrics. For general tips on querying Metric data, see Metric query examples.
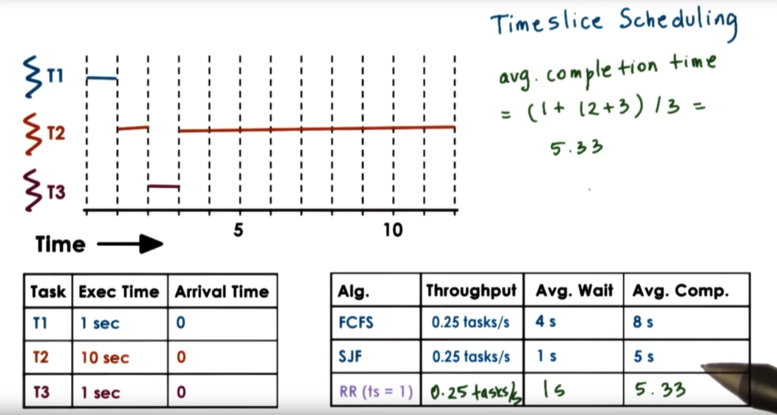
Metric is one of our core data types, and metric timeslice data is stored as this data type.
